Introduction of Artificial Intelligence Acronyms by Alaikas
In the rapidly evolving world of Artificial Intelligence Acronyms by Alaikas play a crucial role. Whether you’re a seasoned professional or a curious beginner, understanding AI acronyms can significantly enhance your comprehension and communication within the industry. These shorthand terms save time, improve efficiency, and are essential in the fast-paced conversations typical of AI discussions.
This article will explore the top 12 best AI acronyms, as curated by Alaikas, and delve into what makes them important. We’ll also examine the significance of these acronyms in various AI applications, how they shape our understanding, and provide insights on how to stay updated with the ever-growing list of AI terminologies.
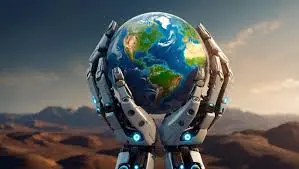
What are AI Acronyms?
Definition and Examples
An acronym is a word formed from the initial letters of a series of words. In AI, acronyms are used to simplify complex terms and concepts, making them easier to reference and communicate. For example, AI itself is an acronym for Artificial Intelligence, a term that represents the simulation of human intelligence by machines.
Why AI Acronyms Matter
AI acronyms matter because they encapsulate complex ideas into simple, memorable terms. This not only aids in communication among professionals but also makes AI concepts more accessible to the general public. In a field where innovation is constant, acronyms help streamline conversations and documentation, allowing for quicker dissemination of new ideas.
The Impact of AI Acronyms on Industry Communication
Enhancing Efficiency
AI acronyms enhance efficiency by providing a common language that professionals across different sectors can understand and use. Whether in academic papers, corporate meetings, or casual discussions, these acronyms make it easier to convey complex concepts quickly and accurately.
Streamlining Technical Discussions
Technical discussions in AI often involve intricate details that can be overwhelming. Acronyms simplify these discussions, ensuring that participants are on the same page. This streamlining is particularly important in collaborative environments where teams need to make quick, informed decisions.
Top 12 Best Artificial Intelligence Acronyms
AI – Artificial Intelligence
Artificial Intelligence, or AI, is the broad concept of machines being able to carry out tasks in a way that we would consider “smart.” It encompasses various subfields, including machine learning, natural language processing, and robotics.
ML – Machine Learning
Machine Learning (ML) is a subset of AI that involves the use of algorithms and statistical models to enable computers to improve their performance on a task through experience. It’s a crucial component of many AI systems, allowing machines to learn from data.
NLP – Natural Language Processing
Natural Language Processing (NLP) is a branch of AI that focuses on the interaction between computers and humans through natural language. The goal of NLP is to enable machines to understand, interpret, and respond to human language in a way that is both meaningful and useful.
CV – Computer Vision
Computer Vision (CV) is an AI field that enables machines to interpret and make decisions based on visual data, such as images or videos. It’s widely used in areas like facial recognition, autonomous vehicles, and healthcare diagnostics.
DL – Deep Learning
Deep Learning (DL) is a subset of machine learning that uses neural networks with many layers (hence “deep”) to analyze various factors of data. It’s particularly effective in image and speech recognition tasks.
RPA – Robotic Process Automation
Robotic Process Automation (RPA) involves using AI to automate routine tasks that are typically performed by humans. This can include anything from processing transactions to responding to customer service inquiries.
AGI – Artificial General Intelligence
Artificial General Intelligence (AGI) refers to a type of AI that is capable of performing any intellectual task that a human can do. While still theoretical, AGI represents the next frontier in AI research.
ASI – Artificial Superintelligence
Artificial Superintelligence (ASI) surpasses human intelligence in all aspects, including creativity, general wisdom, and problem-solving. ASI is often discussed in theoretical and ethical debates about the future of AI.
GAN – Generative Adversarial Network
A Generative Adversarial Network (GAN) is a class of machine learning models in which two neural networks compete with each other to generate new, synthetic instances of data that can pass as real data.
IoT – Internet of Things
The Internet of Things (IoT) refers to the interconnected nature of devices that communicate and share data with each other via the Internet. AI plays a critical role in analyzing and managing the vast amounts of data generated by IoT devices.
ANN – Artificial Neural Network
Artificial Neural Networks (ANNs) are computing systems inspired by the biological neural networks that constitute animal brains. They are the foundation of most deep-learning models.
HCI – Human-Computer Interaction
Human-Computer Interaction (HCI) studies how people interact with computers and to design technologies that let humans interact with computers in novel ways. It is critical to ensure that AI systems are user-friendly and accessible.
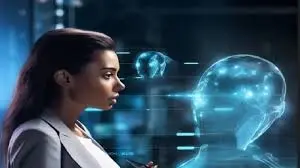
In-Depth Look at Each Acronym
AI – Artificial Intelligence
Overview
Artificial Intelligence encompasses the creation of smart machines capable of performing tasks that typically require human intelligence. These tasks include problem-solving, decision-making, and language translation, among others.
Applications in the Real World
AI is used across various industries, from healthcare, where it aids in diagnostics and personalized treatment plans, to finance, where it helps in fraud detection and automated trading.
ML – Machine Learning
How ML Powers AI
Machine Learning is the driving force behind AI’s ability to improve over time. By analyzing large datasets, ML models can identify patterns and make predictions without being explicitly programmed to do so.
Popular ML Algorithms
Some popular machine learning algorithms include decision trees, support vector machines, and neural networks. Each algorithm has its strengths and is suited for different types of tasks.
NLP – Natural Language Processing
Understanding Human Language
Natural Language Processing enables machines to understand and respond to human language. This is achieved through a combination of linguistic, statistical, and machine-learning techniques.
NLP in Chatbots and Virtual Assistants
NLP powers chatbots and virtual assistants like Siri and Alexa, allowing them to understand user queries and provide relevant responses, enhancing user interaction with technology.
CV – Computer Vision
Machines that See
Computer Vision allows machines to process and analyze visual data, mimicking the human ability to see and interpret the world around us. This is crucial in fields like autonomous driving and security surveillance.
Impact on Healthcare and Security
In healthcare, computer vision aids in medical imaging analysis, improving diagnostic accuracy. In security, it enhances surveillance systems by identifying potential threats in real-time.
DL – Deep Learning
The Next Level of AI
Deep Learning takes machine learning a step further by using complex neural networks with many layers to process data. This approach is particularly effective in tasks like image and speech recognition.
DL in Image Recognition
Deep Learning has revolutionized image recognition, enabling applications like facial recognition, object detection, and even artistic style transfer.
RPA – Robotic Process Automation
Automating Routine Tasks
RPA uses AI to automate repetitive tasks, freeing up human workers to focus on more complex and creative tasks. This is particularly useful in industries like finance, where RPA can handle transaction processing.
RPA in Business Processes
In business, RPA is used to streamline operations by automating processes like invoice processing, customer service, and data management.
AGI – Artificial General Intelligence
The Future of AI
AGI represents the next big leap in AI, where machines can perform any intellectual task that a human can. This level of intelligence could revolutionize industries by automating complex problem-solving.
Challenges in Developing AGI
Despite its potential, developing AGI poses significant challenges, including ethical considerations, computational requirements, and the need for a deep understanding of human cognition.
ASI – Artificial Superintelligence
Beyond Human Intelligence
ASI would surpass human intelligence in every aspect, potentially leading to breakthroughs in science, technology, and medicine. However, it also raises concerns about control and safety.
Ethical Considerations
The development of ASI brings ethical questions to the forefront, such as the potential for machines to make decisions without human oversight, and the impact on employment and society.
GAN – Generative Adversarial Network
Creating Realistic Data
GANs are used to generate realistic images, videos, and other types of data by pitting two neural networks against each other. This technology has applications in everything from art to cybersecurity.
GANs in Art and Entertainment
In the art world, GANs are used to create new pieces of art by analyzing existing works and generating new ones in a similar style. In entertainment, GANs can create realistic digital characters and environments.
IoT – Internet of Things
Connecting the World
The Internet of Things connects devices across the globe, enabling them to communicate and share data. AI is integral to managing and analyzing this data, allowing for smarter and more efficient systems.
IoT and AI Integration
AI and IoT work together to create smart environments, from smart homes that adjust lighting and temperature automatically to smart cities that optimize traffic flow and energy use.
ANN – Artificial Neural Network
Mimicking the Human Brain
Artificial Neural Networks are inspired by the structure and function of the human brain. They consist of layers of nodes, or “neurons,” that process data in a way that mimics human thought processes.
ANN in Modern AI Solutions
ANNs are used in a wide range of AI applications, from voice recognition systems to predictive analytics, making them a cornerstone of modern AI technology.
HCI – Human-Computer Interaction
Bridging the Gap Between Humans and Machines
Human-Computer Interaction focuses on making technology more accessible and user-friendly. In AI, HCI ensures that AI systems are designed with the user in mind, improving usability and adoption.
The Role of HCI in AI Development
HCI is critical in AI development, as it ensures that AI systems are not only powerful but also easy to use and understand. This is particularly important as AI becomes more integrated into everyday life.
How to Keep Up with AI Acronyms
Resources for Learning
Keeping up with AI acronyms can be challenging, but there are plenty of resources available. Online courses, AI glossaries, and industry blogs are great places to start. Engaging with AI communities on platforms like Reddit and LinkedIn can also provide valuable insights.
The Importance of Continuous Learning in AI
AI is a fast-moving field, and staying updated requires continuous learning. Regularly exploring new acronyms and concepts will ensure that you remain knowledgeable and can communicate effectively in the AI space.
Conclusion
Understanding AI acronyms is essential for anyone interested in the field. These shorthand terms make it easier to communicate complex ideas and stay informed about the latest developments in AI. As AI continues to evolve, so too will the acronyms that define it, making continuous learning a necessity.
FAQs
What is the most common AI acronym?
AI (Artificial Intelligence) is the most common acronym in the field, representing the broad concept of machines performing tasks that typically require human intelligence.
How do AI acronyms benefit businesses?
AI acronyms streamline communication, making it easier for businesses to discuss and implement AI technologies quickly and efficiently.
Are AI acronyms difficult to learn?
AI acronyms can seem overwhelming initially, but they become second nature with regular exposure and learning.
Can AI acronyms change over time?
Yes, as AI technology evolves, new acronyms are created, and existing ones may change meaning or usage.
Where can I learn more about AI acronyms?
You can learn more about AI acronyms through online courses, AI glossaries, industry blogs, and by engaging with AI communities on social media.
Be First to Comment